AI-Assisted Emissions Monitor in Hybrid Energy Plants
THE PAST
I was first introduced to mathematical optimisation of energy generation and use in a refinery in the seventies whilst attending a summer placement programme organised by the late Professor Alan Cornish (at Imperial College at the time) whilst in my penultimate year of undergraduate course at Leeds University. I was one of 5 students selected from other UK universities in addition to the 15 students on the undergraduate course at Imperial College. Here, with two other student colleagues from Imperial College, we were able to develop an extensive activity matrix of the Shell South Haven Refinery using linear programming and objective function of energy consumption which included consideration of steam generation in low and high pressure boilers, utility uses of energy in the refinery, heat recovery from condensers, and in-house electricity generation using steam turbine systems and possible seasonal load sharing and selling electricity to the national grid in seasons of low demand. The refinery data- based objective function was optimised by solving the refinery energy activity matrix; using linear regression analysis by the method of Gaussian Elimination and Back-Substitution.
As part of development of the coefficients of our activity matrix, as group members, we interviewed and collected seasonal time-series information of energy demand and supply and activity data in different sections of the refinery. We also recognised that with the arrival of different crudes as feedstock from different parts of the world, there were step changes in the coefficients of the supply and demand and activity intensity in different sections of the refinery. This allowed us to run our optimisation software with different crude specifications for comparison. The refinery management were highly impressed in our ability to provide comparative results of the quantitative effects of crude feedstock chemical specification on energy consumption and use during our end of project presentation as a group.
Most of these data gathering and processing as well as optimisation based on time-series of large data sets can now be done using digital data processing techniques and machine-learning algorithms. Furthermore, we no longer need to monitor data with “after the event” historical accounting records but also dynamically at set time intervals during every day operations. Such easy access to dynamic large data sets of chemical process plant operations now allows for virtual models of operations to be developed using deep-learning and neural network development. I have over the last decade been introduced to these latest digital technologies starting with the development of industrial systems modelling and systems integration design undergraduate course materials as part of a Royal Academy of Engineering Visiting Professor Funded Scheme at the University of Surrey headed by the Exxon-Mobil’s European Head of operations based at the Southampton refinery. Since 2005, I have been involved both with systems R&D and systems modelling teaching materials development in different UK and Universities and organisations abroad; see references.
THE PRESENT
Artificial Intelligence is not only about smart electronic devices, and intelligent robotic machinery development, it has also increasing relevance and value in chemical process plant applications that combine materials recycling with energy harvesting and electricity generation. The contents relating to systems engineering combining chemical with biological engineering applications are being developed as part of university teaching colloquia; see for example Tuzun (2020)[1] for a summary of recent developments in UK, Europe and United States.
Hybrid Energy Process Plants (HEPP) are increasingly used in the production of functional raw materials for secondary industries such as healthcare and cosmetics, pharmaceuticals and household products by recycling from biodegradable biomass such as woodchips, forestry and agricultural residue (see for example, EC Directive (1999/31/EC) and Revised Waste Framework Directive-7 on municipal bio-waste for a typical list of materials) whilst at the same time producing energy for in-plant steam generation as well as producing electricity for the grid. This is a good example of hybrid processing of materials and energy whilst also contributing to agricultural and forestry waste recycling. Such technologies have been successfully scaled to commercial plant operations since 2010 and now plants are operational in a variety of locations in Europe, Canada and the Far East
HE FUTURE
A model digital data processing platform is developed, based on “ Artificial Intelligence (AI) assisted deep-learning” that can be used to identify the environmental emissions patterns from process plants with hybrid energy recovery and energy generation facilities. The non-invasive dynamic monitoring and correlation of particulate, VOCs and other greenhouse gas emissions from semi-batch and continuous process plants is made possible with the use of a Multi-Layer Environmental Perceptron (MLEP) whose operation is depicted in the image below.; see also Tuzun (2020)[2]. The technology is demonstrated to facilitate dynamic environmental impact assessment as well as dynamic corrective action and hazard prevention using MLEP platforms in hybrid energy process plants and combined power plants using process-integrated CO2 capture and storage schemes.
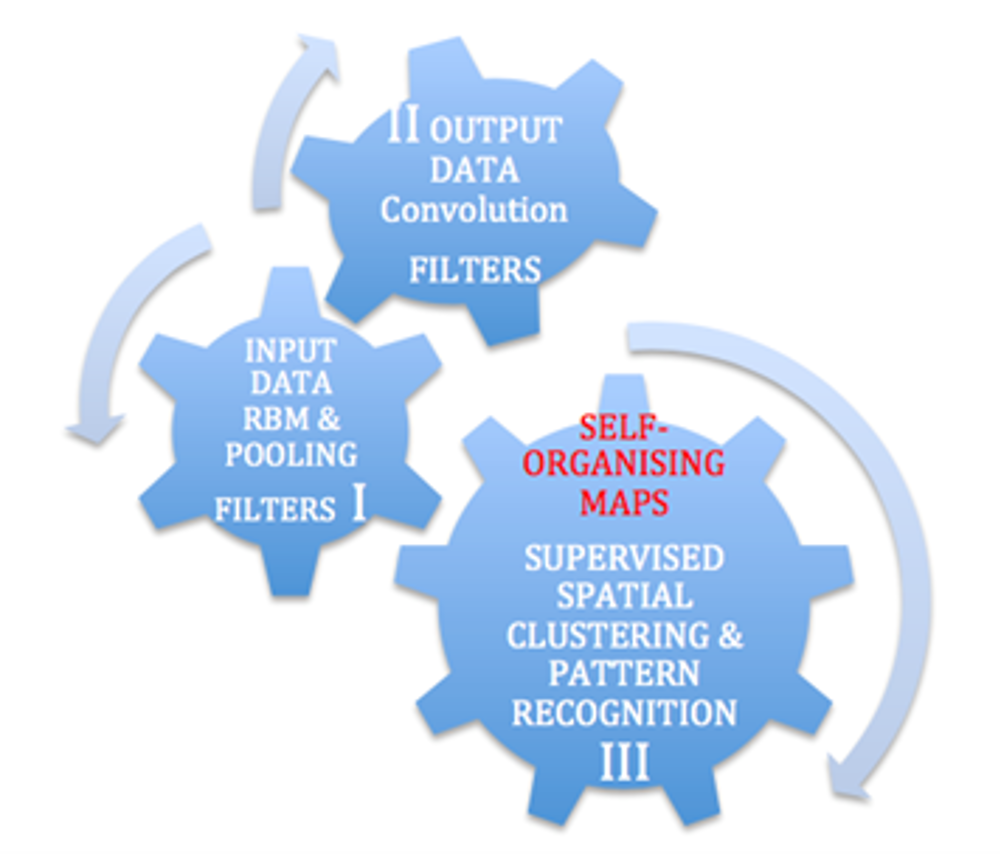
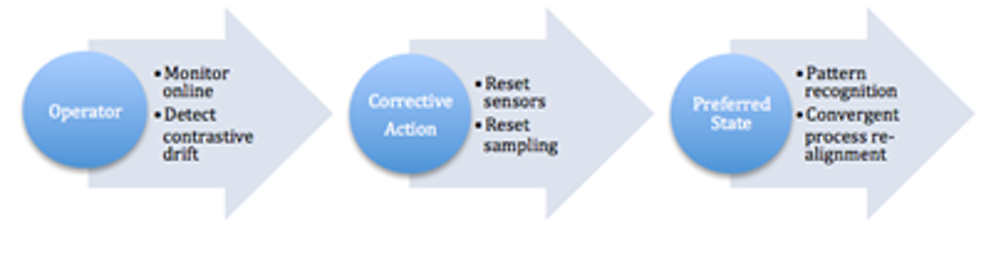
It is appropriate to consider the potential use of the MLEP with industrial plants engaged in (i) synthetic chemicals/materials production, ii) bio-chemicals and biomaterials extraction from natural and agricultural products, and iii) gas and liquid fuels production. Clearly, HEPP’s in the first two categories will have options to consider with regards to combined cycle electricity generation using process heat recovery cycles (as in Fig.1 above) where as the plants engaged primarily in fuel production will increasingly want to exercise options to produce hybrid fuel mixes by dilution of high carbon content of fossil fuels with bio-extracted organics, synthetic organic byproducts and hydrogen; see Tuzun (2020)[1],[2].
[1] Tuzun, U. (2020) Introduction to Systems Engineering and Sustainability Part II: Interactive Teaching of Sustainability Modelling by Systems Integration Design for Chemical and Biological Engineers, Education for Chemical Engineers, Elsevier, 31, 94-104.
DOI: 10.1016/j.ece.2020.04.005
[2]Tuzun, U. (2020) Artificial Intelligence-Assisted Dynamic Control of Environmental Emissions from Hybrid Energy Process Plants (HEPP), Frontiers in Energy Research, 8:179.
DOI: 10.3389/fenrg.2020.00179